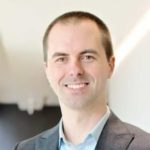
By LUKE OAKDEN-RAYNER, MBBS
I got asked the other day to comment for Wired on the role of AI in Covid-19 detection, in particular for use with CT scanning. Since I didn’t know exactly what resources they had on the ground in China, I could only make some generic vaguely negative statements. I thought it would be worthwhile to expand on those ideas here, so I am writing two blog posts on the topic, on CT scanning for Covid-19, and on using AI on those CT scans.
As background, the pro-AI argument goes like this:
- CT screening detects 97% of Covid-19, viral PCR only detects 70%!
- A radiologist takes 5-10 minutes to read a CT chest scan. AI can do it in a second or two.
- If you use CT for screening, there will be so many studies that radiologists will be overwhelmed.
In this first post, I will explain why CT, with or without AI, is not worthwhile for Covid-19 screening and diagnosis, and why that 97% sensitivity report is unfounded and unbelievable.
Next post, I will address the use of AI for this task specifically.
I’ve been getting a bit upset

Anyone remember Film Critic Hulk? Someone should definitely do a Research Critic Hulk. PUNY SCIENTIST CLAIMS A P-VALUE OF 0.049 IS CLINICALLY MEANINGFUL? HULK SMASH!
I was initially going to write a single post on AI, but as I started reading the radiology literature around CT use in more depth, I found myself getting more and more frustrated. These articles, published in very good journals, are full of flawed designs and invalid conclusions!*
So I have split this post off, and written an article that isn’t about AI at all.
I still think this is relevant for AI-interested readers though, since this is a great example of how a surface level reading of the literature can be really misleading. As always, consult a domain expert before you start building AI models!
CT in Covid-19 is just awful
I’m going to give two arguments here. The first is an unapologetic appeal to authority, for those of my readers who aren’t interested in a discussion on cohort selection, study design, and how research methods determine what sort of claims you can make. The methodology discussion will be in the next section, but you can skip it (and the rest of this blog post) if you want because the experts are unanimous.
The American College of Radiology says “CT should not be used to screen for or as a first-line test to diagnose Covid-19.”
The Royal College of Radiologists says “there is no current role for CT in the diagnostic assessment of patients with suspected coronavirus infection in the UK.”
The Royal Australian and New Zealand College of Radiology says “CT should not be used for routine screening for Covid-19 disease.”
The Canadian Association of Radiologists “recommend against the use of routine chest CT for screening, diagnosis and surveillance of Covid-19 infection.”
There are many more. Boom, argument done. CT sucks.
Technical problems with the radiology literature
If that is a bit flippant, then below is a short technical discussion to explain why the papers are claiming CT is good, and the experts are saying it is bad.
I see two main problems in the literature that suggests high sensitivity for Covid-19 detection with CT:
- severe selection bias, which invalidates all the conclusions
- incredibly permissive diagnostic rules, inflating sensitivity ad absurdum
Selection Bias
I’m going to mostly refer to this paper, since this is the major source the 97% detection claims, which concludes that:
“Chest CT has a high sensitivity for diagnosis of COVID-19. Chest CT may be considered as a primary tool for the current COVID-19 detection in epidemic areas.”
Many sources have picked up on this, both in social media and the traditional outlets.


The problem is that this is totally wrong and not justified by what they showed. The reason for this is called “selection bias”.
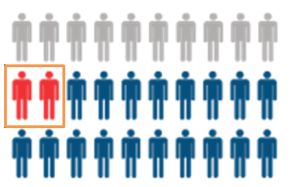
When the patients you select for your study (orange box) aren’t representative, you have selection bias.
Selection bias is when the subset of cases you have tested (called the cohort) is significantly different than the people you want to apply the results to (the population). In a screening setting, you would like to apply the test to anyone who is suspected of Covid-19 (having a cough, sore throat, contact with infected person etc). In the diagnostic setting, you might do a CT after some other tests (like blood counts or similar) to exclude other things first, but you will still be testing a lot of people.
In the study they analysed a retrospective cohort of 1014 patients from Tongji hospital who had both CT scans and viral PCR tests (the current gold standard). The idea is that we can see which patients with viral PCR positive tests within a few days of the scan also had CT features that would allow us to diagnose the disease. Seems fine, right?
Here is the cohort diagram:

The question you need to ask is “which patients were these? Were they from the general population of patients who might have Covid-19?”
The answer is “no, these are patients in the hospital that got referred for CT scans.”
The cohort is therefore biased because the patients a) had a reason to be at hospital, likely because they were hospitalised, and b) had a reason to get an expensive, time-consuming test (the CT scan).
The authors never comment on how these patients were selected (which isn’t really acceptable IMO), but we can infer that the cohort is biased because the radiology literature and the clinical literature are reporting different things. The radiology studies say that nearly 100% of patients have CT changes, but the clinical reports says that 81% of cases of Covid-19 either have no pneumonia or mild pneumonia. While the use of terms like “mild” and “severe” infection are pretty rubbery, we should recognise that one common definition is that patients with mild infection don’t go to hospital. If that is the definition used here, 4 out of every 5 Covid-19 patients won’t be represented in this cohort at all!
Not only are 80% of all Covid-19 patients potentially not included in the study, but these excluded patients are also specifically those who are less likely to have CT findings. One study suggests that over 50% of patients who have Covid-19 infection will have a negative CT study in the first few days of infection (likely a decent proxy for “mild infection”). Another study showed that only 50% of asymptomatic patients had changes on CT.
Put this all together, and we could imagine an extreme scenario where the study reports a sensitivity of 97%, but the actual sensitivity for screening is below 50% (and possibly as low as 20-30%).
This was so strange that I thought I was missing something as I read these studies, so I asked radiology Twitter for input. The overall position among the TwitterRadis was pretty similar to mine; there was extreme skepticism that these results reflect the true population.

The only thing that is >95% certain is that my tweets will have typos in them. Blame the 5-day old newborn who was keeping me awake
Blinding and misleading metrics
The second problem relates to the metric choice itself. Sensitivity is a great metric, since it tells us “of people with the disease, how many will the test detect?” This is exactly what we care about with Covid-19 screening.
But sensitivity has a seesaw effect with another metric, specificityh m(false positives you get). As sensitivity goes up, specificity goes down. There is a trade-off, and how much you favour one metric or the other will be determined by the clinical setting. In a screening scenario, it can be reasonable to aim for high sensitivity, so you miss as few cases as possible, at the expense of a lower specificity (we can accept a higher level of false positives).

The trade-off between sensitivity and specificity is often plotted as a ROC curve.
What is often not appreciated, however, is that you can turn the dial all the way. It is always possible to achieve 100% sensitivity (top right corner of the curve); you simply say that everyone has the disease.
This is an absurd approach, since a) it means a whole lot of people without the disease are getting treated, and b) it completely invalidates the test. Why even do the test if you don’t need to look at it?
With that in mind, let’s look at another “CT is highly sensitive for Covid-19 detection” paper. This study, in the same high-tier journal, claims that the sensitivity of CT is 98% vs a sensitivity of 70% for viral PCR. They say:
“Our results support the use of chest CT for screening for COVD-19** for patients with clinical and epidemiologic features compatible with COVID-19 infection particularly when RT-PCR testing is negative.”
With a statement as strong as that, surely they are saying that they have evidence that CT can be applied broadly? They even mention patients with epidemiological features of infection … that is to say, folks who have been exposed but don’t have symptoms.
Here is the patient cohort diagram:

We can see we are again looking at only hospitalised patients who underwent CT, so we are likely to be overestimating the sensitivity due to selection bias.
But I noticed something else in this paper too – they show us the images for some cases, and some of the images are a bit weird. The image below, which was reported to contain “atypical features of Covid-19” (specifically “small peripheral linear opacities bilaterally”), was a massive red-flag for me.

Well, they must be small, because that looks like a normal CT chest
Now, maybe that image was unrepresentative or included by error, but they show this one as well, with a handy arrow:

According to this paper, tiny random opacities are now diagnostic of Covid-19.
I’m getting snarky here, this is only 2 images out of the dozen or so in the paper, but would anyone like to guess what percentage of healthy patients have a tiny vague opacity or scattered basal interstitial markings?

Old memes are good memes.
The point here isn’t that this test will have low specificity. It will, but as I said, that can be acceptable for a screening test. Rather, the problem is that the way they have performed the study throws the sensitivity results into doubt. It is trivial to achieve high sensitivity if you call any abnormality on an image a positive result. We could have equally high sensitivity on chest x-rays too, because almost every study ever has some degree of atelectasis (which could, in theory, be a ground glass opacity and therefore Covid-19).

Look at that minor left basal atelectasis. Must be Covid.
This also highlights a major problem with the whole study design. They don’t actually say how they identified the features of disease (again, pretty unacceptable), so I can only assume they had some radiologists look at the images post-hoc (commonly called a “reader study”). If that is the case, then how did this study actually work? Did they say “here are confirmed cases of Covid-19, tell me if you can see any features of the disease”?
In radiology research we always try to blind readers to the actual outcomes, because otherwise they will be biased by the known answer; doctors will be actively trying to find evidence that supports the already proven diagnosis. They aren’t cheating on purpose, this is a known subconscious bias that affects their performance.
Blinding is the incredibly important, because in real life the readers don’t know who has the disease. If you don’t blind, your results will not reflect reality.
Even if they didn’t tell the doctors that these cases all had Covid-19, how long do you think it will take for the doctors to twig that almost every case is diseased? A reader study without negative examples is, effectively, unblinded.
It is worth noting the the first study I discussed (in the section about selection bias) was appropriately blinded and had negative cases, so the blinding issue may or may not be a problem, but at the very least I am concerned that the authors were overly permissive in what criteria they used to define CT-positive results.
Summary
In my opinion, the studies that have reported high sensitivity for CT imaging are fatally flawed. They only report results on highly biased hospital populations, and it is likely that the reporting rules used were too broad to be a fair estimate of the real-world use of the tests.
There have been several studies which showed more plausible results. A large study in NEJM of hospitalised patients (still a biased cohort) in China showed that, in practice, about 18% of CT scans were normal at admission. This is real-world evidence, as they used the radiology reports to determine the CT diagnosis rather than having radiologists read the scans post-hoc.
The cruise ship study I already mentioned is nice as well, since this is a group of exposed people who were all quarantined and comprehensively screened. They found that 50% of asymptomatic Covid-19 positive patients had no CT changes, and (similar to the above NEJM study), around 20% of symptomatic patients were also CT negative.
These results are markedly different from the optimistic early reports in the radiology literature, and the reason for this is clear. They looked at the holistic population rather than a small biased sample in hospitals, and (at least for the NEJM study) they used the actual clinical reports to assess the presence of CT changes, rather than only looking at positive cases without negative controls through the retrospectoscope.
Rather than 97% or 98%, the overall sensitivity of CT for the detection of Covid-19 infection is probably below 50%, and is almost certainly much worse than PCR testing. Thus, as all of the expert panels and committees have said, CT imaging has no role in the screening or primary diagnosis of Covid-19.^
*this is not unique to the radiology literature, but there have been quite a few studies of questionable value in good radiology journals.
**typo in the final line of the conclusion is reproduced from the source. This isn’t a comment on the authors, more a reflection of the reduced editorial oversight that comes with any disaster. I don’t doubt that the need to get papers out (with both good and questionable motivations***) is responsible for some of the problems raised above.
***I’d be remiss to not mention the questionable motivations, although I am not aiming this at the authors of the papers discussed here or the journal editors, some of whom I know. There is a metric crap-ton of terrible research on covid coming out. The writing and publication of rubbish science is heavily motivated by the near infinite citations on offer, the unlimited altmetrics, the slavering media chasing after new and controversial content, and even political agendas. And, next blog post, in AI in particular there are millions of dollars on the table for even vaguely believable claims, as governments want to look like they are doing something rather than nothing, and AI always looks good in a press release.
^that is not to say that CT scanning has no role at all. We do imaging for a wide range of reasons, but that is a different discussion. The papers claim that we can use CT for screening and diagnosis, and that appears unsupported by the evidence.
Luke Oakden-Rayner is a radiologist in South Australia, undertaking a Ph.D in Medicine with the School of Public Health at the University of Adelaide. This post originally appeared on his blog here.
The post CT scanning is just awful for diagnosing Covid-19 appeared first on The Health Care Blog.
from The Health Care Blog https://ift.tt/3drBOBz
via IFTTT
No comments:
Post a Comment