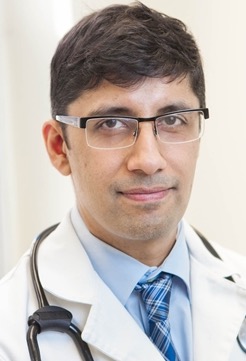
By ANISH KOKA, MD
Something didn’t seem right to epidemiologist Eric Weinhandl when he glanced at an article published in the venerated Journal of the American Medical Association (JAMA) on a crisp fall evening in Minnesota. Eric is a smart guy – a native Minnesotan and a math major who fell in love with clinical quantitative database-driven research because he happened to work with a nephrologist early in his training. After finishing his doctorate in epidemiology, he cut his teeth working with the Chronic Disease Research Group, a division of the Hennepin Healthcare Research Institute that has held The National Institute of Diabetes and Digestive and Kidney Diseases (NIDDK) contract for the United States Renal Data System Coordinating Center. The research group Eric worked for from 2004-2015 essentially organized the data generated from almost every dialysis patient in the United States. He didn’t just work with the data as an end-user, he helped maintain the largest, and most important database on chronic kidney disease in the United States.
For all these reasons this particular study published in JAMA that sought to examine the association between dialysis facility ownership and access to kidney transplantation piqued Eric’s interest. The provocative hypothesis is that for-profit dialysis centers are financially motivated to keep patients hooked to dialysis machines rather than refer them for kidney transplantation. A number of observational trials have tracked better outcomes in not-for-profit settings, so the theory wasn’t implausible, but mulling over the results more carefully, Eric noticed how large the effect sizes reported in the paper were. Specifically, the hazard ratios for for-profit vs. non-profit were 0.36 for being put on a waiting list, 0.5 for receiving a living donor kidney transplant, 0.44 for receiving a deceased donor kidney transplant. This roughly translates to patients being one-half to one-third as likely to get referred for and ultimately receiving a transplant. These are incredible numbers when you consider it can be major news when a study reports a hazard ratio of 0.9. Part of the reason one doesn’t usually see hazard ratios that are this large is because that signals an effect size that’s so obvious to the naked eye that it doesn’t require a trial. There’s a reason there are no trials on the utility of cauterizing an artery to stop bleeding during surgery.
But it really wasn’t the hazard ratios that first struck his eye. What stuck out were the reported event rates in the study. 1.9 million incident end-stage kidney disease patients in 17 years made sense. The exclusion of 90,000 patients who were wait-listed or received a kidney transplant before ever getting on dialysis, and 250,000 patients for not having any dialysis facility information left ~1.5 million patients for the primary analysis. The original paper listed 121,000 first wait-list events, 23,000 living donor transplants and ~50,000 deceased donor transplants. But the United Network for Organ Sharing (UNOS), an organization that manages the US organ transplantation system, reported 280,000 transplants during the same period.
The paper somehow was missing almost 210,000 transplants.
Eric proceeded to re-run a rough version of the paper’s analysis, and came up with numbers that were markedly different than the initial analysis. The analysis still favored the not-for-profit centers, but the hazard ratios were now 0.8 – 0.9.
Eric felt compelled to act, but he felt awkward. He was, after all, an epidemiologist employed by a for-profit dialysis chain poking holes in a paper from academics that made for-profit dialysis companies look bad. On the recommendation of an associate editor at JAMA, he began a correspondence with the senior author of the paper, who expressed confidence in her team’s analysis despite the basic and serious flaws that were obvious with regards to event rates. The author did offer to place the code for the paper on an open-source platform for Eric to examine.
It didn’t take long to figure out what the error was. The USRDS database that Eric knew so well includes provider numbers – numeric values that map to CMS certification numbers that label the dialysis facilities. The code merged the patient roster database which contained the certification number of the dialysis facility at initiation with the waitlist database that contained its own certification number of the hospital at which the patient was first listed.
The researchers used a commonly used statistical software to do their analysis called SAS. A particularly unique feature of SAS learned the hard way for many is that it doesn’t handle merges where columns may contain the same name. It doesn’t even throw up a warning when this happens. It simply overwrites one column with the other.
Since almost all transplants in the United States take place at not-for-profit centers, the waitlist database consists almost entirely of not-for-profit facilities. When this database was combined with the larger end stage kidney database, the merged database ended up enriched with the non-profit facilities associated with the waitlisted patients. Ultimately, too many wait-list events and transplants end up being mapped to not-for-profit hospitals, and other events that should be mapped to a dialysis facility, regardless of profit status, are excluded. The result is a paper with exaggerated hazard ratios in favor of not-for-profit facilities.
Six months later JAMA retracted and republished the paper with corrected numbers. The results still suggested a statistically significant benefit in favor of non-profits, but the size of the effect was much attenuated with an absolute cumulative 2.6% lower rate of referrals and kidney transplantation at for-profit dialysis centers over 5 years. These conclusions are fragile for a number of reasons. This study, for instance, attributes a patient’s waitlist/transplant outcome to the very last dialysis facility the patient was associated with. In epidemiology speak, this means the causal inference authors are trying to draw between for-profit status and good transplant outcomes is subject to time-varying confounding. As an example, if one is seeking an association between testosterone levels and risk of a heart attack, using the last testosterone level available would be a poor way of doing this study because testosterone levels are known to vary over time. The same applies to dialysis facilities. Patients change dialysis facilities, and facilities may change their profit status if ownership changes. Eric did his own sensitivity analysis (personal communication), and this time changed the profit status indicator to the dialysis facility on record after 3 months of dialysis as opposed to the last dialysis facility – the difference in outcomes seen is even further attenuated. The choice of the last dialysis facility prior to waitlisting is particularly interesting given that most waitlist activity happens in the first 2 years of getting on dialysis. Clinical reality would suggest that what would be of most interest to physicians and patients alike would be the discovery of dialysis facilities that are not aggressive in referring patients for transplant early after patients initiate dialysis. But beyond the somewhat esoteric discussions of how much exposure and when exposure occurs to dialysis facilities, waitlist and transplantation rates are arguably most dependent on a number of factors that have little to do with the dialysis facility. In reality transplant rates vary widely by factors like geography and the number of locally available transplant centers that are well outside of the dialysis facility’s control. In summary, the republished effect sizes are so small as to be considered remarkably uncertain when all the other confounding factors in an observation analysis are considered.
The original editorial that ran with the first (now retracted paper) noted:
Assuming the findings of these studies … are valid and unbiased, it might be reasonable to infer that for-profit dialysis organizations have systematically and disproportionately focused their resource investments to prioritize the delivery of dialysis services while paying less attention to ensuring patients receive transplants.
While the qualifier here about “assuming the findings of the study were valid” is appreciated, the fundamental rotting core of the problem is that authors of the editorial have no business writing an editorial about a topic they clearly have no domain expertise in. The authors and journal would have everyone believe that the error is confined to a technical problem related to database merges. The real problem, of course, runs far deeper.
The authors, peer reviewers, and editorial writers for one of the most prestigious journals in the world didn’t notice that the original manuscript was missing almost 200,000 transplants that took place during the study period. This is akin to MSNBC’s Brian Williams and New York Times editorial board member Mara Gay nodding and smilling as they discussed a tweet that complained Michael Bloomberg wasted $500million on campaign advertising, when he could have given a $1million check to every one of 327 million Americans.
One may excuse journalists in 2020 for being unplugged from math and reality, but the bar should be a little higher for reviewers and editorial writers of the top scientific journals in the land. Part of the problem may be that the researchers and the editors (we don’t know who the reviewers were) aren’t nephrologists and certainly aren’t epidemiologists with a deep understanding of kidney disease. Instead, what we have are public health dilettantes and population health scientists who specialize in number crunching, but have a poor understanding of the data they are analyzing.
It would be of little consequence if this group of academics was confined to discussing their results at cocktail parties, but this particular group of outcomes researchers help guide federal government policy on reimbursement. It’s like giving a 12 year old keys to a Ferrari. What we’re supposed to get is an intelligent policy path paved by evidence, but the trouble is that this isn’t a dispassionate objective group seeking truth wherever it may lie. Rather, their forte is data crunching and writing papers to confirm strong biases held within the public health community at large.
It’s well known that schools of public health have a certain politics attached to them that overwhelmingly favor a progressive ideology. The bias, in this case, is an outgrowth of a strongly held public health belief that health care as a for-profit enterprise is a problem to be rectified. It’s difficult to see where ideology ends and science starts in this arena. The public health community at this point has generated mountains of evidence for the superiority of a not-for-profit system in delivering health. Unfortunately much of the evidence is generated by those with a strong pre-existing bias on the matter. Every research project becomes an exercise in confirming that bias. It’s normally hard to make this out, but the JAMA retraction does provide a nice window into how “science” in this case is far from value-neutral.
Despite the fact that re-running the analysis resulted in much smaller absolute differences that are likely to disappear if slightly different methodologic paths are taken, basically the same editorial ran with the republished article. If anything, the authors of the editorial attempt to make the small absolute difference seen even more meaningful by translating the fragile 2.5% difference over 5 years into the potential for 45,000 fewer waitlists over the 16 year study period. This is standard operating procedure. Take a large database. Plumb it for a politically correct conclusion. When an analytic path inevitably leads to a small but statistically significant conclusion, apply the small difference across the large denominator to emphasize the importance of the small finding.
As an example, take another JAMA paper: A large retrospective study of hospitalists by gender demonstrated patients treated by female physicians had a statistically significant 0.43% difference in mortality, the head of the Harvard School of Public health could have noted that the methodology was a bit crude. There are no patients that are only taken care of by male or female physicians, so the study assigned physician gender based on the gender that took care of the patient during a hospitalization most of the time. Since men and women physicians are now relatively equally split, most of the time translated to a particular physician being responsible, on average, is 51.1% of the total time ‘spent’ with the patient during a particular hospitalization. So an extra 1.1% of time spent with patients by female physicians is enough to generate a difference in mortality. Never mind that the teams of people like nurses, respiratory therapists, techs, and medical residents with varying gender mixes take care of patients as well in hospitals. Even if this was somehow true how does one possibly apply this to the individual physician? Should we embark next on studies to generalize the effect of Hindus on patient care, and then with some small result in hand, make generalizations about the care of all Hindu physicians?
If any opinion is to be rendered on the basis of this particular study comparing male and female physicians, it should channel the difficulty of coming to any hard conclusions because of the limitations of the dataset and the small effects found. Instead, we have the senior physician on the study write an opinion piece that suggests 32,000 fewer deaths per year are to be gained by making male physicians more like their female counterparts in some unknown way, and that the outcome gap makes the longstanding and controversial physician gender pay gap even more “unconscionable”.
It is little surprise then that the retracted and republished dialysis study runs with essentially the same editorial with the same conclusions regarding for-profit status without any reference to how the results materially changed. A passing reference in the correction notice to a senior epidemiologist who works at a for-profit dialysis enterprise is made and is a testament to how academia functions. Dominance in the academic hierarchy is frequently established not by the bubbling of the best ideas and evidence to the top, but by discrediting those with opinions that don’t come from the academic cabal. Eric Weinhandl, the study authors are quick to point out, worked for a for-profit dialysis organization.
So what?
I would argue that it was precisely because he was in this role that he chose to delve deeper into the original paper. The perceived conflicts here are a distraction. The key ingredients in this mess are large dollops of bias combined with a frightening lack of domain expertise in every step of the making of this sour-tasting stew.
This isn’t to malign bias in research. After all, bias is what allows intelligent hypotheses to be created. The fact that there are health services researchers that think the for-profit enterprise is a bad model in healthcare is a good thing. The issue is that the overwhelming majority of the public health community engaged in generating evidence believes this. This leads to an echo chamber with no opponents that would allow the sharpening of arguments. The problem isn’t that Eric Weinhandl was working for a for-profit organization, it is that there are no Eric Weinhandl’s that appear to be part of the academic tapestry. Combine this with poor methodological analysis that is, in part, derived from a shallow understanding of subject matter studied and we find ourselves with poor science and garbage results. Sadly, there is no reason to think this isn’t a systemic problem that infects the entirety of the public health research enterprise.
The schools of public health are production factories for graduates with an understanding of the tools one can use to analyze data. This doesn’t mean they understand the limitations of the data or the disease they happen to be investigating. What they do end up doing has been artfully described by statistician Andrew Gelman as taking the garden of forking paths: when researchers embark on projects with strong pre-existing biases and consciously or unconsciously choose analytic paths that confirm their biases.
Ideally, public policy research would be a self-correcting enterprise where ideological diversity combined with subject matter expertise allowed for robust critique and analysis. When this doesn’t happen, the conclusions and accompanying editorials are written before the data is even analyzed. The research, then, is just for show.
Anish Koka (@anish_koka) is a for-profit cardiologist in Philadelphia and co-host of a healthcare-focused podcast, The Accad & Koka report. He still maintains he’s a nice guy.
from The Health Care Blog https://ift.tt/2Zz959q
via IFTTT
No comments:
Post a Comment